À l’ère du Big Data, l’intelligence collective des êtres humains permet de générer de nombreuses données pour résoudre certains des principaux problèmes de l’humanité. De même, elle permet d’analyser certaines données plus efficacement que les algorithmes informatiques. Découvrez la relation étroite entre intelligence collective et Big Data.
Intelligence collective : définition
La notion d’intelligence collective désigne une intelligence de groupe, ou une intelligence partagée émergeant de la collaboration, des efforts collectifs ou de la compétition entre plusieurs individus. Celle-ci permet de prendre des décisions par consensus. Les systèmes de vote, les réseaux sociaux, et les autres méthodes permettant de quantifier l’activité de masse peuvent être considérés comme des intelligences collectives.
Ce type d’intelligence apparaît comme une propriété émergente de la synergie entre le savoir offert par les données, les logiciels, le hardware informatique, et les experts de domaines spécifiques, permettant de prendre de meilleures décisions au moment opportun. Plus simplement, l’intelligence collective résulte de l’association entre les humains et les nouvelles façons de traiter l’information.
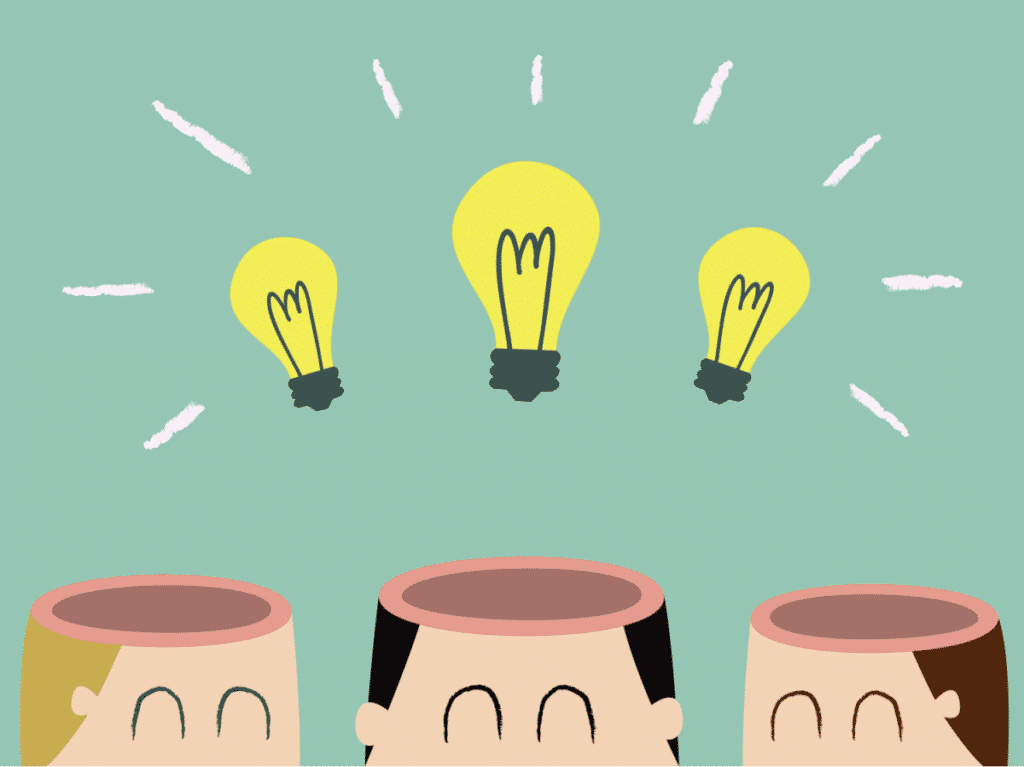
Un concept très répandu
Le concept d’ intelligence collective dispose sociologie, en informatique, mais aussi dans le domaine du business. Pour Pierre Lévy, il s’agit d’une forme d’intelligence distribuée de façon universelle, qui s’améliore constamment, se coordonne en temps réel et découle sur une mobilisation efficace de compétences. Le fondement et l’objectif de cette forme d’intelligence sont la reconnaissance mutuelle et l’enrichissement des individus plutôt que le culte des communautés hypostasiées. Aux yeux de Pierre Lévy et Derrick de Kerckhove, elle désigne la capacité des réseaux de technologies informatiques à approfondir le bassin collectif de savoir social en étendant simultanément la portée des interactions humaines.
Elle contribue fortement à la transition du savoir et du pouvoir de l’individu vers le collectif. Selon Eric S.Raymond et JC Herz, l’intelligence Open Source permettra éventuellement de générer des résultats supérieurs au savoir généré par des logiciels propriétaires développés au sein d’entreprises. Pour Henry Jenkins, elle est une source alternative de pouvoir médiatique. Ce dernier critique notamment les écoles et les systèmes éducatifs, faisant la promotion de la résolution autonome de problèmes et de l’apprentissage individuel. Il reste cependant hostile à l’apprentissage via ce biais. Malgré tout, comme Pierre Lévy, il considère qu’elle est essentielle pour la démocratisation, car liée à la culture basée sur le savoir et alimentée par le partage d’idées. De fait, cette dernière contribue à une meilleure compréhension d’une société diverse.
Origine du concept d’ intelligence collective
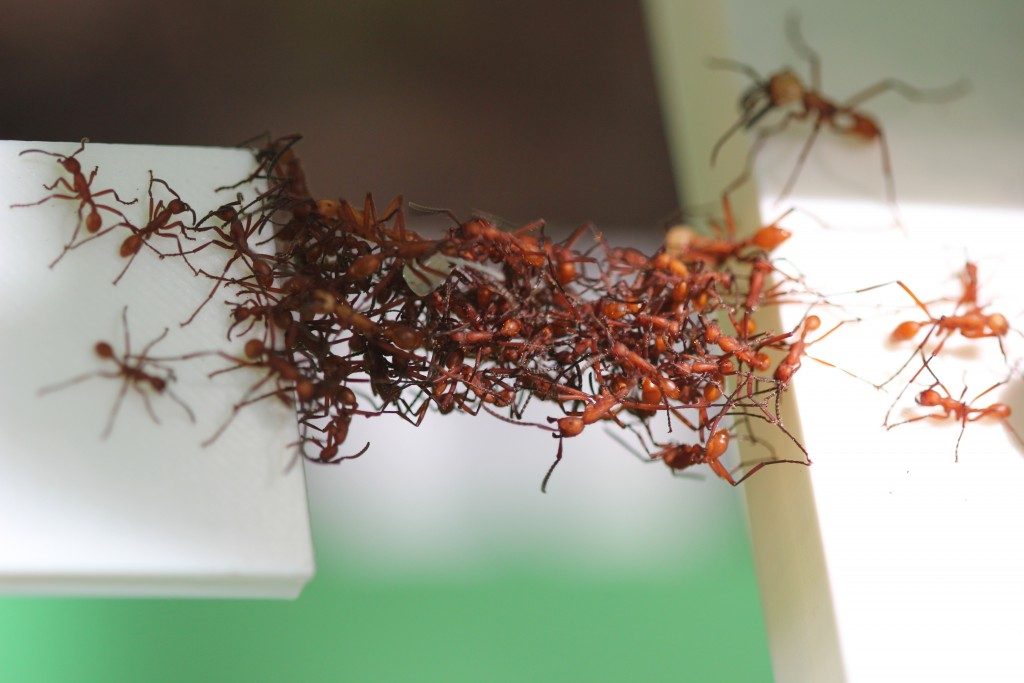
Le concept d’intelligence collective remonte à 1785. C’est à cette époque que le Marquis de Condorcet souligne que si chaque membre d’un groupe a de plus fortes probabilités de ne pas prendre une décision correcte, la probabilité pour que le vote majoritaire de ce groupe soit la décision correcte augmente avec le nombre de membres du groupe. Il s’agit du théorème du jury.
Un autre précurseur de ce concept est l’entomologiste William Morton Wheeler. Selon ses propos datés de 1911, des individus apparemment indépendants peuvent coopérer au point de devenir un organisme unique, une intelligence collective. Le scientifique a perçu ce processus collaboratif en observant les fourmis, agissant comme les cellules d’une entité individuelle.
En 1912, Émile Durkheim a identifié la société comme la seule source de pensée humaine logique. Selon lui, la société constitue une intelligence supérieure, car elle transcende l’individu dans l’espace et le temps. En 1962, Douglas Engelbart a établi le lien entre l’intelligence collective et l’efficacité d’une entreprise. Selon lui, trois personnes travaillant ensemble à la résolution d’un problème seront efficaces bien plus que trois fois par rapport à une seule personne.
Intelligence collective à l’ère du Big Data
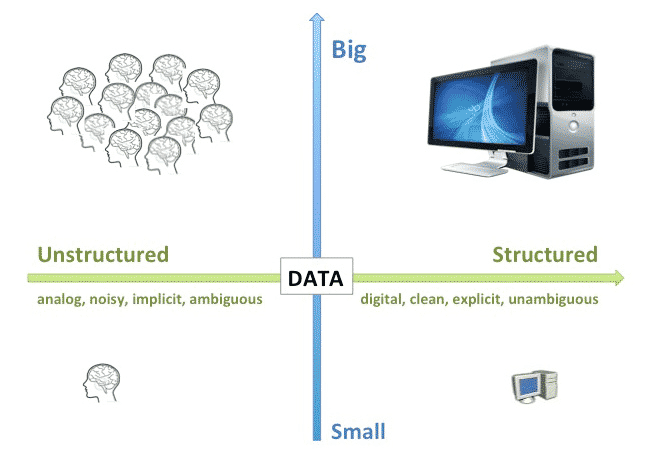
À l’ère du Big Data, de nombreuses entreprises ont tendance à chercher des réponses à leur question à l’endroit où elles sont faciles à chercher, plutôt qu’à l’endroit où il est probable de les trouver. En réalité, les probabilités pour qu’un groupe de recherche Big Data découvre une information utile dépend du type de données disponibles. Les données structurées, numériques, explicites et lisses seront plus facilement traitées par des ordinateurs, tandis que les données non structurées, analogues et ambigües, ont davantage de sens pour les cerveaux humains.
Toutefois, pour un humain comme pour un ordinateur, plus les ensembles de données sont conséquents, plus la puissance de calcul nécessaire est importante. Dans le cas des données structurées, des ordinateurs plus puissants feront l’affaire. En revanche, pour les données non structurées, il sera indispensable de s’en remettre à l’intelligence collective de plusieurs cerveaux humains.
Si l’objectif est de prédire le futur, une approche statistique du Big Data s’avère particulièrement bancale. En effet, les données disponibles s’enracinent nécessairement dans le passé. De fait, ces données peuvent certes permettre de prédire des situations similaires à celles du passé, par exemple pour une ligne de produits mature sur un marché stable, mais deviennent inutiles pour les prévisions liées à de nouveaux produits ou des marchés bouleversés.
Intelligence Collective : exemple d’applications
Voici quelques exemples de situations pour lesquelles les prédictions réalisées par l’intelligence collective s’avèrent plus utiles que les prévisions menées par le Big Data :
Marchés bouleversés : l’ intelligence collective en entreprise
Au milieu des années 2000, la demande mondiale en produits laitiers a soudainement été multipliée par trois en l’espace de quelques mois. Après une décennie de stabilité, les producteurs de produits laitiers ne pouvaient plus se baser sur les modèles prédictifs basés sur les données. Par conséquent, les acteurs de l’industrie se sont appuyés sur la collectivisation des agricultures, plus proches des consommateurs, pour mieux comprendre et modéliser les facteurs de cette nouvelle demande.
Nouveaux produits
Il y a quelques années, Lumenogic a collaboré avec une équipe de chercheurs en marketing pour réaliser une prédiction de marché au sein d’une entreprise du Fortune 100, en se focalisant sur de nouveaux produits. Cette méthode prédictive s’est révélée plus pertinente que les prévisions basées sur les données dans plus de 67% des cas, en réduisant la moyenne d’erreurs d’environ 15%, et en réduisant la portée des erreurs de 40%.
Élections politiques
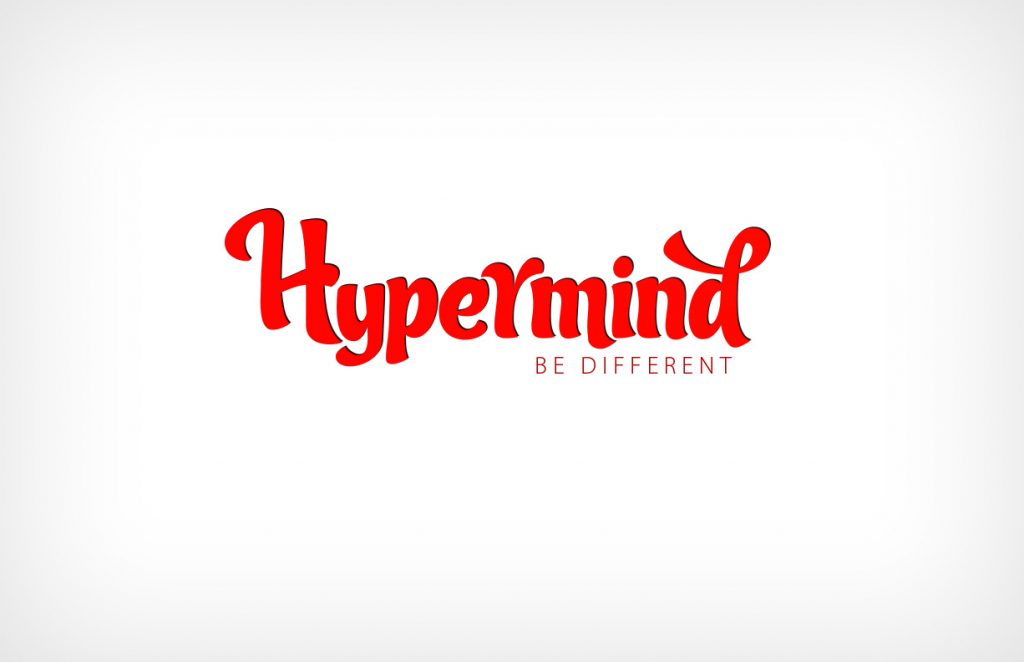
Au cours des 20 dernières années, les marchés de prédiction sont devenus célèbres pour leur capacité à surpasser les sondages dans le domaine des prédictions des résultats électoraux. En novembre dernier, dans le cadre des élections présidentielles américaines, l’intelligence collective des traders de Hypermind a dépassé tous les modèles de prédictions statistiques data-driven mis en place par les géants du média. L’explication est simple. Elle est capable d’agréger de nombreuses informations non structurées sur ce qui rend chaque élection unique, à un niveau inaccessible pour les algorithmes statistiques, aussi sophistiqués soient-ils.
Malgré le flot actuel de données structurées, en pleine effervescence, il est essentiel de garder en tête que le monde regorge de données non structurées. Seul l’esprit humain y trouve un sens. Ainsi, si vous vous retrouvez à chercher des réponses en vain en explorant le Big Data, rappelez-vous qu’elle pourrait remédier au problème.
L’ intelligence collective et les Open Data pour comprendre les épidémies
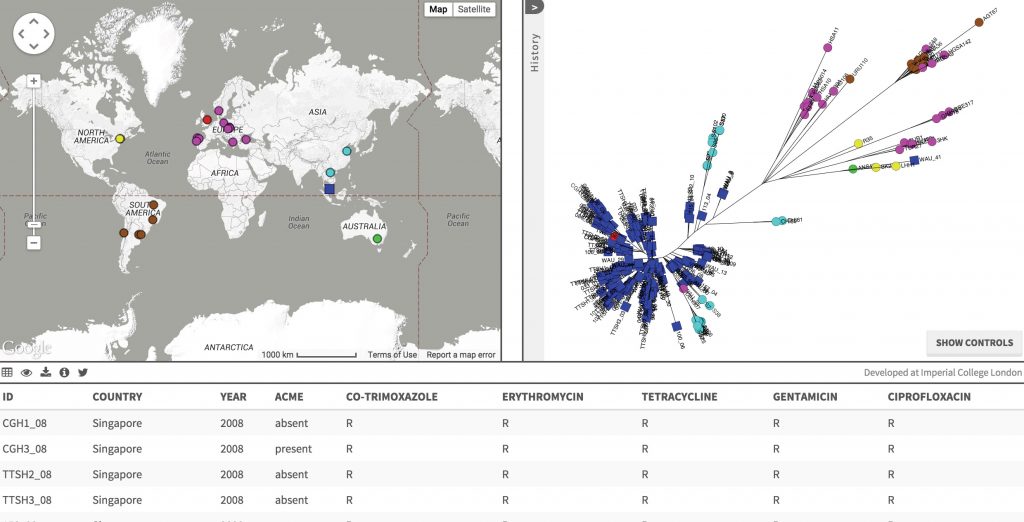
Les chercheurs du Welcome Trust Sanger Institute et de l’Imperial College London ont développé Microreact. C’est une plateforme gratuite de visualisation et de suivi en temps réel des épidémies. Cet outil a notamment été utilisé pour surveiller les épidémies d’Ebola, de Zika, et de microbes résistant aux antibiotiques. L’équipe a collaboré avec la Microbiology Society pour permettre à tous les chercheurs du monde de partager leurs dernières informations au sujet d’épidémies.
Jusqu’à présent, les données et les informations géographiques sur les mouvements et évolutions des infections ou des maladies ont été cantonnées à des bases de données inaccessibles au public. Les chercheurs ont dû s’en remettre aux informations publiées dans des articles sur la recherche, parfois expirés. Ils contenaient uniquement des visuels statiques présentant une petite partie de la menace épidémique.
Système Microreact : faciliter le partage de données
Le système Microreact est basé sur le cloud, et combine le pouvoir des données ouvertes et de l’ intelligence collective du web pour proposer une visualisation et un partage de données mondiales en temps réel. Tout un chacun peut explorer et examiner les informations avec une vitesse et une précision inédite. Cet outil peut jouer un rôle primordial dans la surveillance et le contrôle des épidémies comme Zika ou Ebola.
Les données et les métadonnées se téléversent sur Microreact depuis un navigateur web. Elles peuvent ensuite être visualisées, partagées et publiées depuis un lien web permanent. Le partenariat avec Microbial Genomics permet au journal de créer des données à partir de publications prospectives. Ce projet promeut la disponibilité ouverte et l’accès aux données, tout en développant une ressource unique pour les professionnels de la santé et les scientifiques du monde entier.
Les travaux du Dr Kathryn Holt et du professeur Gordon Dougan illustrent à merveille la façon dont Microreact peut démocratiser les données génomiques et les insights qui en résultent. Ces derniers ont récemment publié deux articles sur la distribution mondiale de la bactérie typhoïde et la propagation épidémique résistante aux médicaments. Ils ont aussi publié leurs données directement sur Microreact pour aider d’autres chercheurs à développer d’autres travaux.
En publiant ainsi ces données sur Microreact, les chercheurs ont assuré la pérennité des données. Ils ont permis à d’autres d’apprendre de leurs travaux. Ceux-ci ont utilisé les informations en guise de base de comparaison ou de fondation pour de futurs projets. Microreact permet également aux chercheurs individuels de partager des informations à l’échelle mondiale et en temps réel.
L’intelligence collective pour résoudre les principaux problèmes de l’humanité
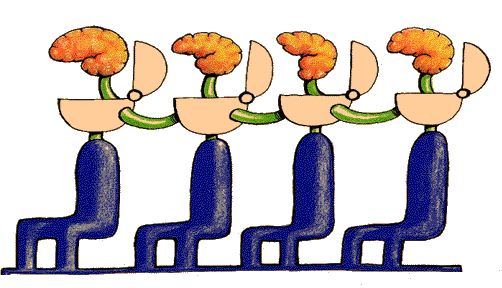
D’ici 2050, l’humanité devra vraisemblablement faire face à de nombreux problèmes. La montée des océans, le réchauffement climatique, la pénurie de ressources sont quelques-uns des défis que nous devrons relever. Pour y parvenir, nous pourrons et devrons nous en remettre à l’intelligence collective.
Grâce à l’essor des forums internet, des groupes de recherche, des pages wiki, des réseaux sociaux et de la blogosphère, de nouvelles méthodes de résolution de problèmes ont émergé. Les scientifiques perçoivent désormais internet comme un groupe de recherche commun. Ce mode d’apprentissage et de communication que l’on peut catégoriser en intelligence collective permet de déterminer des consensus de nombreux esprits pour trouver une réponse à des challenges complexes.
Gérer le changement climatique par ce prisme
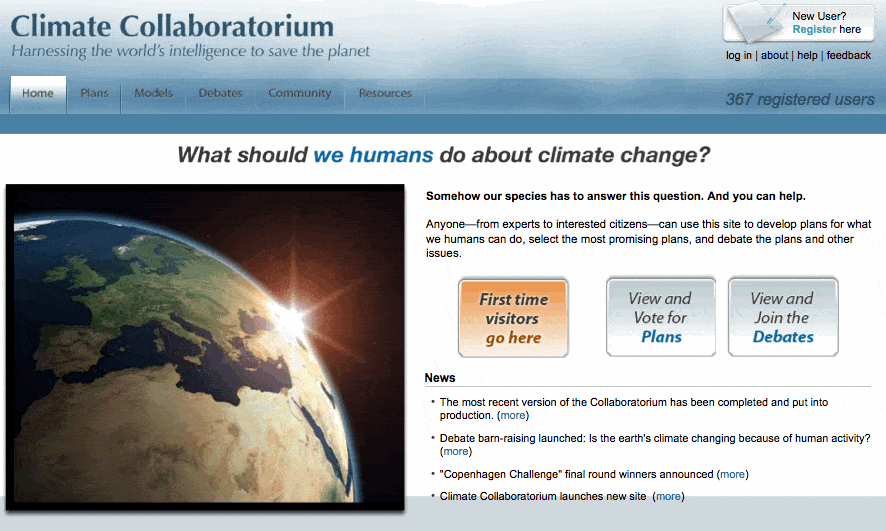
Le centre pour l’intelligence collective du MIT développe un forum en ligne baptisé Climate Collaboratorium. Ce forum se présente comme un modèle informatique en perpétuelle évolution. Il représente l’atmosphère de la planète Terre et des systèmes humains. Il est alimenté par des salles de discussion scientifiques en ligne. Toutes les variables et les facteurs liés au climat, comment l’environnement, les interactions avec les humains et l’écologie sont inclus dans ce modèle évolutif.
Le professeur Thomas W. Malone, fondateur du centre, compare le Collaboratorium au Manhattan Project. Ce dernier a développé la bombe atomique pendant la Deuxième Guerre mondiale. La différence est que le Collaboratorium vise à résoudre un problème concernant tous les êtres humains. Grâce aux nouvelles technologies, à commencer par internet, il est possible de fédérer beaucoup plus de personnes que pendant la Deuxième Guerre mondiale.
En fin d’année 2014, le Climat CoLab comptait 33000 membres en provenance de plus de 150 pays. La NASA, la World Bank, l’Union of Concerned Scientists, de nombreuses universités et autres agences gouvernementales s’impliquent dans le projet. Le projet a pour but de cumuler toutes les possibilités des êtres humains pour lutter contre les changements climatiques. Les solutions sociales, politiques, économiques, et les solutions d’ingénierie sont passées en revue.
Développer de meilleurs jets avec l’intelligence collective
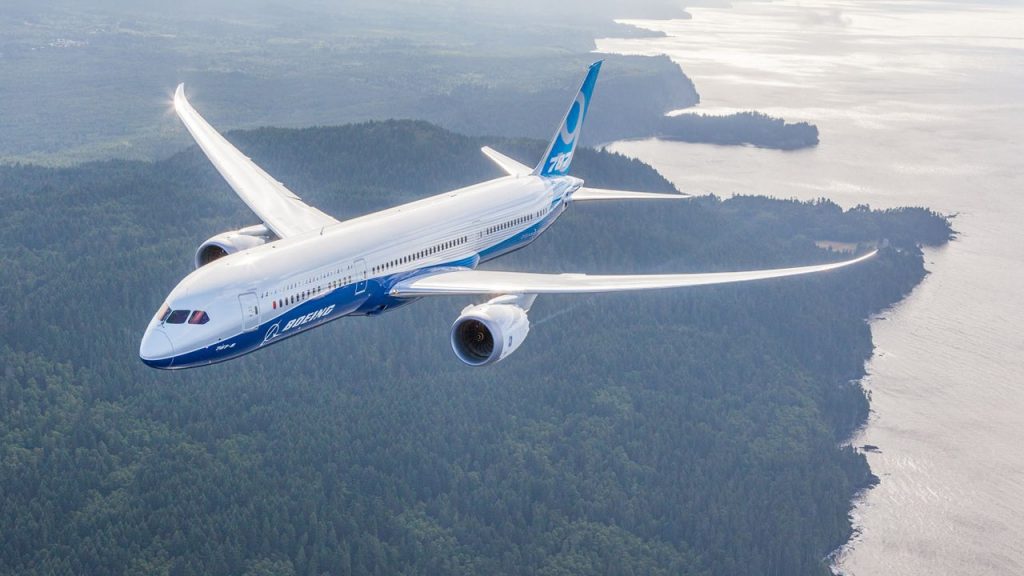
Boeing utilise le potentiel créatif de l’intelligence collective pour concevoir des jets. Le 787 Dreamliner a été créé en collaboration avec plus de 1000 partenaires proposant chacun leurs idées pour créer l’avion ultime. Ce jet, commercialisé en 2011, reprend des éléments du design du 777 combinés avec des matériaux composites comme le plastique renforcé à la fibre de carbone. Cela représente environ 50% de la structure principale pour remplacer l’aluminium. Ce jet a instauré un nouveau standard en termes d’efficience et de confort.
Afin d’accélérer le processus de design à moindre coût pour cet avion novateur, Boeing a décidé de s’en remettre à ses fournisseurs. Le Global Collaborative Environment (GCE) lie tous les membres de l’équipe de design du 787. Auparavant, Boeing a conçu 70% de l’avion. Puis, il a laissé ses 43 fournisseurs et de nombreux autres sous-traitants en provenance de 24 pays collaborer sur 135 sites. Pour l’avancée du projet, ces partenaires ont abandonné leurs systèmes de design assisté par ordinateur respectifs pour le langage et le format communs du système Catia V5 de Boeing. Grâce à ce programme standardisé de communication de données et de design, Boeing a réduit sa documentation de 2500 à 20 pages.
L’intelligence collective, les groupes de recherche en ligne, le stockage cloud et le Big Data sont les nouveaux moteurs de la pensée créative. Les problèmes complexes et critiques auxquels l’humanité est aujourd’hui confrontée nécessitent de déployer des solutions plus rapidement qu’autrefois. C’est pourquoi l’usage de ces technologies est désormais indispensable.
Seoul Innovation Challenge, un projet d’urbanisme basé sur l’intelligence collective
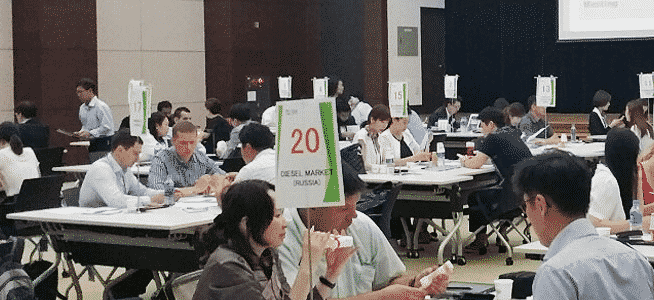
Le Seoul Innovation Challenge vise à faire appel à l’intelligence collective pour résoudre les problèmes urbains rencontrés par Seoul dans les domaines de la sécurité, de l’environnement et du trafic. Ce challenge s’est déroulé pendant 200 jours, et était ouvert aux citoyens, aux étrangers, aux entreprises et aux universités. Les maîtres mots sont la coopération, l’innovation, et l’ouverture. Lorsque quelqu’un suggérait une idée novatrice sur la plateforme, tous les participants se sont lancés dans un processus collaboratif auprès de 100 mentors professionnels dans les 7 mois suivants.
L’étape préliminaire a eu lieu en juillet 2017. 32 projets furent choisis. Pendant les trois mois suivants, les idées ont été développés. L’étape finale a lieu à la fin du mois de novembre. Un soutien sera apporté aux 32 projets en vue d’une commercialisation. L’enregistrement de propriété intellectuelle, la démonstration et la mise en place de partenariats, ainsi que la recherche d’investisseurs seront assistées. Il était possible de s’inscrire à ce projet d’intelligence collective sur le site officiel.
L’intelligence collective peut-elle surpasser l’intelligence artificielle ?
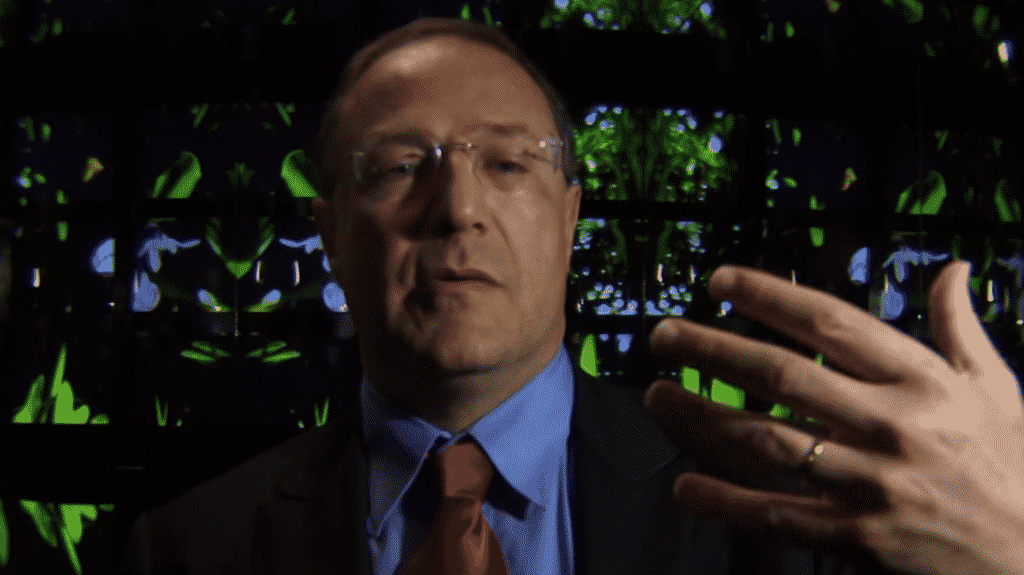
Le philosophe français Pierre Lévy, spécialisé dans l’intelligence collective, développe depuis 2015 un logiciel permettant d’analyser les données en provenance des réseaux sociaux. Il veut comprendre les motivations réelles des humains. Ce logiciel permet de transformer automatiquement les mots de divers langages dans un hyper-langage algorithmique symbolique intitulé « Information Economy MetaLanguage ».
Selon l’auteur de « L’intelligence collective : Pour une anthropologie du cyberespace » , elle se retrouve initialement dans la nature. Cependant, grâce au langage et à la technologie, celle associée aux humains s’avère largement supérieure. Pourquoi ? Parce qu’elle repose sur la manipulation de symboles. Nous sommes entrés dans l’ère de la manipulation algorithmique des symboles.
Pour le philosophe, cette forme collective d’intelligence s’oppose à l’intelligence artificielle. Son objectif n’est pas de rendre les ordinateurs plus intelligents, mais d’utiliser les ordinateurs pour rendre les humains plus intelligents. Pour ce faire, Lévy compte créer un système de catégorisation universel aussi souple que le langage naturel. Il servira à classifier les innombrables données disponibles sur le web. La relation sémantique détermine l’orchestration des données. Ce système permettra l’émergence de nouvelles idées. En somme, l’IEML permet de connecter les idées grâce à l’informatique. Le philosophe considère qu’internet représente déjà une forme d’intelligence de ce type, mais souhaite y ajouter une notion de réflexion.
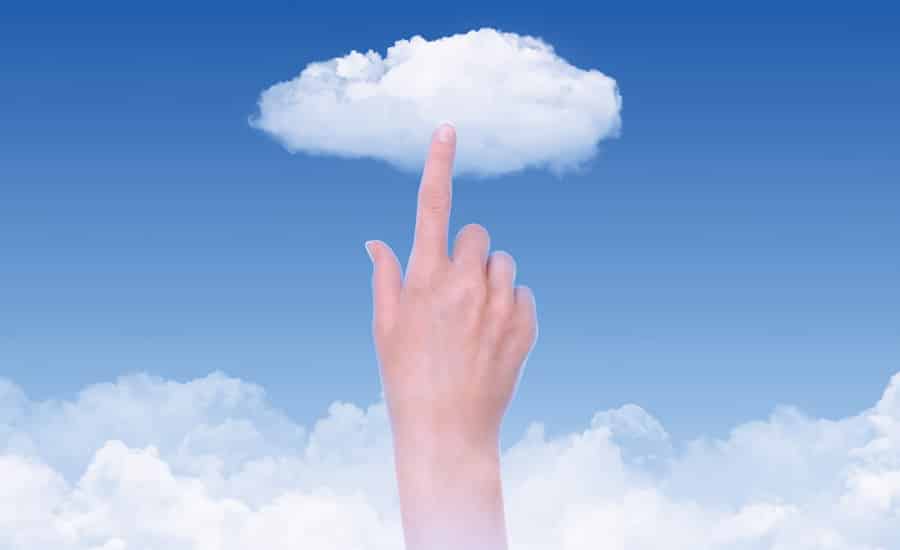
Conscient que ce système pourrait permettre à de grandes entreprises comme Apple et Google, ou à des agences gouvernementales comme la NSA d’accéder à une quantité d’informations d’une ampleur inédite, Pierre Lévy précise toutefois que son objectif est d’offrir le pouvoir de l’information au peuple. Tout comme les activistes de la Silicon Valley dans les années 70, qui souhaitaient permettre à tout un chacun d’accéder à l’informatique, ce philosophe souhaite donner au peuple la possibilité d’analyser et de donner sens aux données disponibles sur internet.
Intelligence collective : un logiciel crée par Pierre Levy
Pour ce faire, Pierre Lévy compte s’appuyer sur deux outils. Le premier outil est le langage IEML, et le second est le software qui implémente ce langage. Les entreprises distribuent ce logiciel de manière ouverte et gratuite. Il dispose de la troisième version de GPL. Enfin, tous les changements apportés àl’IEML doivent être totalement transparents.
Certes, tout le monde ne peut pas contribuer au dictionnaire IEML, car il s’agit d’un savoir spécialisé. Des compétences linguistiques, ou des connaissances mathématiques, sont indispensables. Cependant, tous le monde peut créer de nouvelles étiquettes. Le philosophe considère que c’est le maximum que l’on puisse faire pour permettre aux gens d’accéder à la liberté. On ne peut forcer les gens à être libres, mais on peut leur donner tous les outils nécessaires pour s’émanciper.
Le Big Data favorise-t-il la formation à l’Intelligence collective ?
La formation à l’ intelligence collective fait de plus en plus d’émule. Les entreprises veulent faire en sorte que leurs employés mettent en commun leurs ressources cognitives afin de progresser vers un même but qui peut généralement se résumer à l’augmentation du profit de l’entreprise. En ce sens, certaines organisations prennent part à des séminaires d’équipe. Il s’agit de favoriser cette réflexion collective, puisqu’elle basée sur l’obtention d’une cohésion de groupe.
Le Big Data couplé à l’intelligence artificielle peut faciliter cette phase de formation. En effet, les algorithmes de correspondance peuvent trouver les points communs entre des membres d’une équipe et ainsi favoriser les interactions fructueuses. La bonne entente n’est pas le seul élément d’une bonne intelligence collective. Il faut également savoir qu’un individu travail sur le même projet que vous. Dans une grande entreprise répartie à différents endroits de la planète, cela n’est pas forcément évident. Un lac de données réparties dans un Cloud et les outils d’accès en entreprise peuvent donner cette information.
Dans une optique de formation, le Big Data apporterait dans l’idéal des moyens de mesurer la progression des membres d’un séminaire. Il s’agirait d’évaluer les interactions dans le groupe, et même de prédire a quel point sera amélioré la collaboration dans l’entreprise.
Source : https://blog.hypermind.com/2015/01/28/the-role-of-collective-intelligence-in-the-age-of-big-data/
Cet article Intelligence collective et Big Data : définition, exemples en entreprise a été publié sur LeBigData.fr.